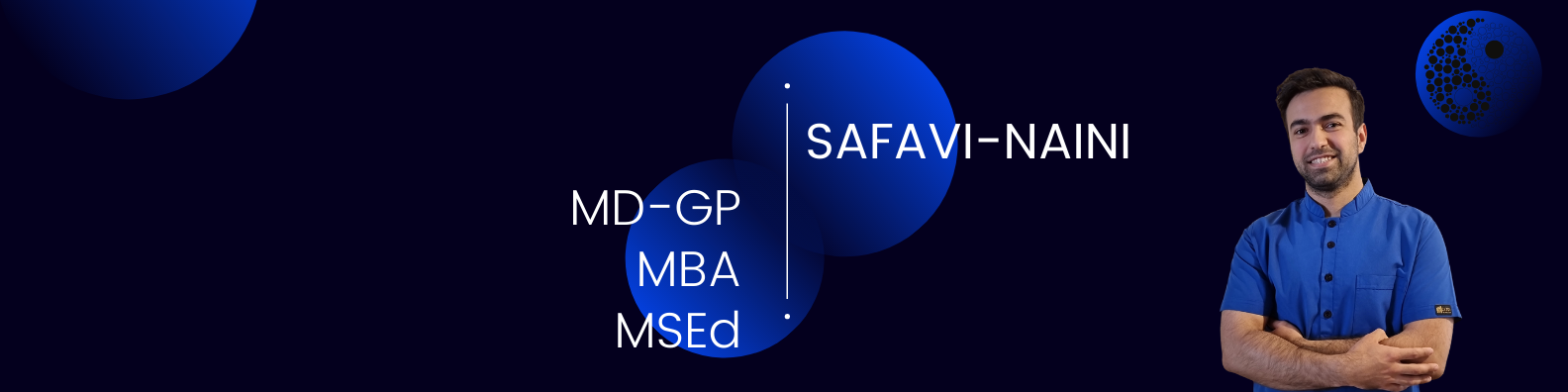
I am an MD-MBA graduate specializing in precision medicine, with a combined three years of both industrial and academic research experience. During the latter part of my medical schooling, I dedicated myself to numerous research initiatives in COVID-19 and internal medicine, striving to understand the defining characteristics and warning signs of various diseases.
Post-medical school, I embarked on a research fellowship from 2021-2023 at RIGLD. During this tenure, I led three notable projects: 1- A collaborative effort spanning multiple institutions to develop a comprehensive model for the diagnosis and prognosis of pancreatic cancer. 2- The 3D reconstruction of perianal fistulae. 3- The detection of lesions in capsule endoscopy images.
Outside of research, I’ve had the privilege to mentor over 10 medical students. It’s a point of pride for me that several of these individuals have become pioneers in their respective fields. Their achievements stand as the most gratifying testament to my endeavors.
My primary research interest lies in integrating artificial intelligence into routine healthcare practices. I am particularly passionate about harnessing its potential to improve the quality of life for patients suffering from digestive diseases. I believe that patient data, rather than being seen as a mere asset, is a profound responsibility. My mission is to use this data to reciprocate benefits directly back to the patients from whom it originated.
Why We Should Shift to personalized Medicine and Data-Driven Decision making
In the past, the limitation of data and infrastructures urged us to ground our medical practice on the “general population and assumptions.” For example, if a drug worked on 80% of the population, we assumed it would work on all. If a patient comes with cancer, we will stage his(er) disease with flow charts created from “general population” data. Although these guidelines are essential to start the practice, a clinician will start using his(er) clinical scene as he gains experience. If we want to translate it to personalized medicine language, the first approach uses lower dimensions, while the latter results from clinical pattern recognition and higher data dimensions.
With advancements in computation infrastructure and data availability, we can now promote precision medicine. It is then that we can find out if you are among 20% of people who do NOT get the effect of your drug. We can find your risk of complications, diseases, and cancer recurrence, personalized for you. However, cross-disciplinary areas, like AI deployment in medicine, need team members who understand computational and clinical fields. And that is my goal, to bridge this gap and improve patient health through personalized medicine.